Exploring the Evolution of Data Management: Beyond Warehouses
Written on
Chapter 1: The Changing Landscape of Data Management
As organizations increasingly adopt Data Warehouses and, to some extent, Data Lakes, newer concepts like Data Lakehouses and Data Meshes are emerging. Are these the next generation of data management platforms?
To clarify, Data Warehouses and Data Lakes serve distinct roles in the realm of Big Data storage. A Data Lake functions as an expansive repository for raw data whose future applications are not yet defined. Conversely, a Data Warehouse is designed to house structured and filtered data that has been processed for specific objectives [1]. For further insights, click here.
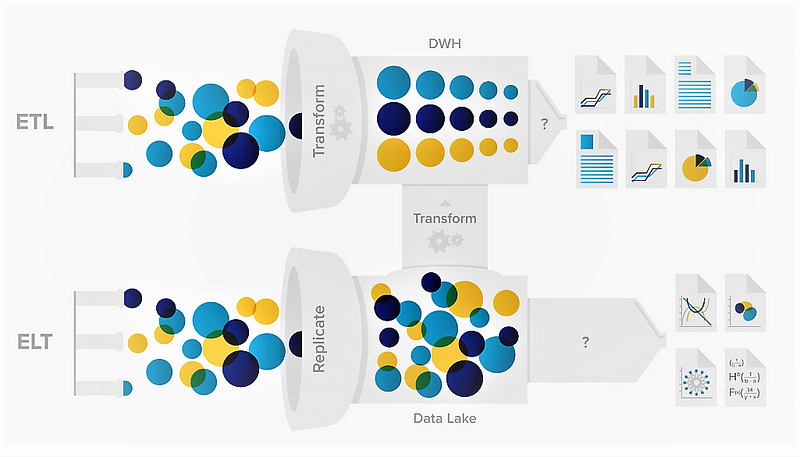
Data Warehouse vs Data Lake — Source: Big2Smart [2]
Similar to how Data Warehouses are built on the foundations of Data Lakes, Data Lakehouses aim to merge the two concepts. However, the creation of a Data Lakehouse involves more than just fusing a Data Lake with a Data Warehouse; it encompasses the integration of both along with specialized storage solutions to facilitate unified governance and seamless data movement [3].
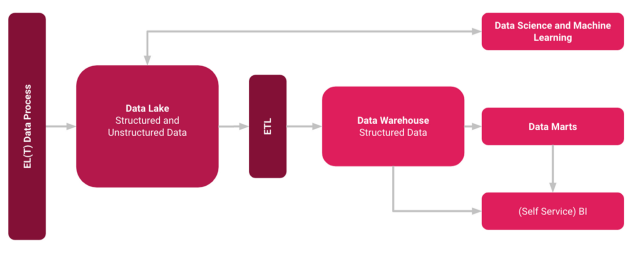
Data Lakehouse Concept — Image from Author
The advantages of implementing a Data Lakehouse include [4]:
- Separation of data storage from processing, enhancing scalability.
- Utilization of open standardized storage formats and interfaces.
- Capability to handle diverse data types, ranging from unstructured to structured formats.
- Support for varied workloads, including data science, machine learning, SQL, and analytics.
- Comprehensive streaming capabilities, allowing real-time data applications without needing separate systems.
- Reduced time-to-value when compared to traditional Data Warehouses.
A Data Mesh approach further refines the Data Lakehouse paradigm. Notably, the concept of a Data Mesh emphasizes a novel organizational viewpoint rather than solely focusing on technical solutions. Here are four key principles to consider when establishing a Data Mesh organization [4]:
- Domain-oriented Decentralized Data Ownership and Architecture: A Data Mesh should cater to individual business units, potentially leading to the development of one or more Data Lakehouses.
- Data as a Product: This architecture enables teams focused on specific domains to manage the entire data lifecycle.
- Self-serve Data Infrastructure as a Platform: Users can independently access data through self-service BI tools, while data scientists can utilize the same datasets for model development.
- Federated Computational Governance: Data should be securely backed up and distributed according to a defined role framework, with data catalogs enhancing usability.
I trust this overview clarifies the relationships among Data Warehouses, Data Lakes, Data Lakehouses, and Data Meshes, as well as how these systems complement one another. It’s important to note that traditional architectures are not entirely replaced but are rather modernized and enhanced. The Data Mesh primarily serves as an organizational strategy, integrating technology to foster data-driven business practices.
Sources and Further Reading
[1] Talend, Data Lake vs. Data Warehouse (2022)
[2] Big2Smart, Data Lake vs. Data Warehouse: what’s the Difference and which is the Best Data Architecture? (2022)
[3] AWS, What is a Lake House approach? (2021)
[4] Stefan Koch, Können Lakehouses einen Paradigmenwechsel anstossen? (2021)
Chapter 2: Understanding the Differences
To grasp the distinctions between these data storage solutions, check out the following videos:
This video explains the differences between databases, data warehouses, and data lakes, highlighting their unique features and purposes.
An in-depth look at the variations between databases, data warehouses, and data lakes, providing clarity on why these differences matter.