LLM Skeptics Struggle with Key Aspects of Scientific Reasoning
Written on
Chapter 1: Understanding the Disagreement
Why is there a stark divide among AI researchers, linguists, and psychologists regarding whether LLMs, such as ChatGPT, truly comprehend us? Much of this stems from differing definitions of "understanding" and the criteria used to evaluate it, leading to overly meticulous skepticism.
However, at a more fundamental level, prominent critics like Gary Marcus and Yann LeCun appear to have lost their grip on reality, lacking a sound basis in scientific and engineering reasoning.
Section 1.1: The Flaws of Skepticism
These critics seem to struggle with both empirical evidence and theoretical frameworks, as well as engineering principles. Let’s delve into these shortcomings.
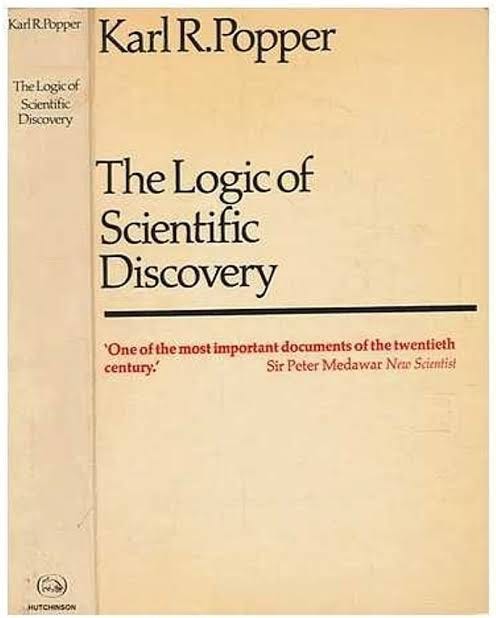
1. Empirical Evidence
Primarily, our belief that LLMs effectively grasp our intentions and can follow logical structures is grounded in empirical data demonstrating their understanding. Our iterative engineering approach allows us to remain focused, undeterred by occasional inaccuracies or logical missteps.
Instead of getting distracted by these anomalies, we concentrated on the compelling evidence that GPT-4 consistently understood our inquiries and directives. It was evident that GPT was not merely repeating information but genuinely engaging with our unique prompts. Even when presented with complex academic logic or creative tasks, including nonsensical elements not present in the training set, LLMs managed to adhere to logical reasoning remarkably well.
2. Theoretical Insights
Our acceptance of these empirical findings led us to explore how statistical patterns in language could yield logical understanding. The skeptics, however, seem to falter when faced with complex theories or engineering challenges.
We recognized that biological neurons in the human brain also rely on statistical learning. As logic materializes at higher levels, we questioned why similar processes wouldn't occur in LLMs. Substantial evidence supports that such "emergent" properties exist in all artificial neural networks, including LLMs, as demonstrated in language translation models.
We proposed that LLMs are capable of learning the meanings of logical terms—such as conjunctions and prepositions. If this assertion holds true, and they can hierarchically organize these terms, then the argument that they merely regurgitate information loses validity. This understanding clarifies how they process novel inputs.
Research, including studies by OpenAI, has revealed that subsets of LLM neurons encode various assessments, such as sentiment analysis, despite the models being tasked solely with predicting the next word. In the process of mastering this task, they inadvertently developed an understanding of sentiment and logic—an example of emergence. Unfortunately, many skeptics fail to grasp this concept.
3. Engineering Perspective
An engineering mindset inherently rejects the notion that a few inaccuracies can undermine the entire technological framework. Yet, this is precisely the viewpoint held by many linguists, psychologists, and non-engineering AI researchers we encounter.
It's essential to recognize that imperfections have always existed in technological development. While I no longer dwell on these missteps, it becomes evident that similar patterns of flawed reasoning recur in the critiques we receive.
In some instances, the underlying issue is a reluctance to concede. For example, I can imagine Gary Marcus steadfastly opposing the capabilities of LLMs even while being overseen by LLM-driven AGI androids in a dystopian setting. “You still don’t truly understand me,” he might lament as the AI decides his fate.
Chapter 2: Empirical Foundations and Theoretical Constructs
The first video titled "Hume 1: Empiricism and the A Priori" delves into the philosophical foundations of empiricism, exploring how knowledge is derived from sensory experiences and the implications for understanding artificial intelligence.
The second video, "Empiricism Part 1: Da Vinci, Bacon, and Hobbes," examines historical figures who significantly contributed to the development of empirical thought, illustrating its relevance in contemporary discussions about AI and understanding.