The Challenges of Transitioning into Data Science Careers
Written on
Understanding the Realities of Data Science
During my initial experience reviewing resumes for data science interns, I experienced a wave of imposter syndrome. Many of these recent graduates possessed the same, if not greater, technical expertise as I did. It led me to question my qualifications as a "senior" data scientist when fresh talent seemed to match my skill level.
My early interactions with these candidates reinforced my concerns; they excelled in technical assessments and demonstrated proficiency in mathematics. I found myself pondering my own value in the field.
However, working closely with these interns and junior data scientists revealed a significant truth: resumes and technical interviews often fail to capture the full spectrum of necessary skills.
The Missing Element
The primary gap in most candidates' profiles isn't technical know-how. Instead, it's the ability to bridge the gap between a business objective and the corresponding algorithm. It’s crucial to recognize which tools to deploy based on the problem at hand—knowing when to use a hammer versus a screwdriver.
Business challenges are inherently intricate. They seldom align perfectly with technical solutions. A deep understanding of both the business issues and technical tools is vital for determining which aspects can be simplified or approximated.
An Illustrative Example
To clarify, let’s consider a practical example. In my time working in healthcare, the ultimate aim of predictive analytics was to foresee which patients would require hospitalization. Successfully identifying these individuals could lead to preventive measures, benefiting both the patients and the organization financially. At first glance, this appears to be a straightforward classification challenge, right?
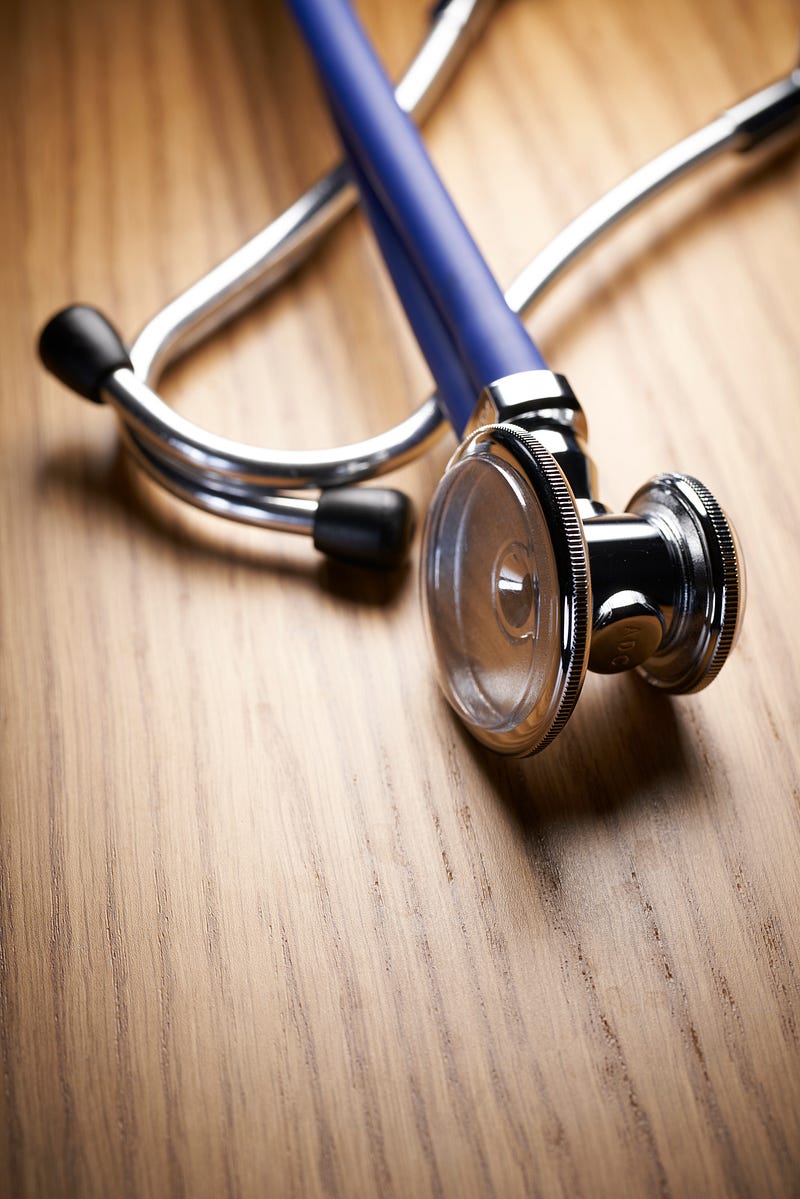
Photo by Bill Oxford on Unsplash
However, it was far more complicated. Numerous underlying questions arose, including:
- How early do we need to identify patients before hospitalization?
- In efforts to prevent hospitalization, how should we interpret predictions based on immutable high-risk factors (e.g., age)?
- Should our focus be on preventable hospitalizations or those we can most likely influence?
- What are the repercussions of false positives versus false negatives?
- If deemed financially viable, do we have unlimited resources, or is there a cap on the number of patients we can reach?
- What level of accuracy is required for the model to be deemed valuable?
Many of these inquiries lack straightforward answers. Some necessitate subjective judgment, while others demand a reevaluation of the problem itself, often requiring a balance between business objectives and technical methodologies.
In my experience, collaborating with business stakeholders is invaluable for addressing these questions; however, technical expertise is essential for identifying the right inquiries.
A Fresh Perspective on Resumes
Acquiring the judgment and business acumen necessary to effectively translate a business challenge into a technical solution takes time and experience. Unfortunately, these qualities are often hard to discern on a resume, yet it becomes evident when someone lacks these competencies.
Candidates with resumes solely showcasing Kaggle projects, Coursera classes, or online tutorials highlight their technical abilities without demonstrating the practical application of these skills to real-world business problems. While these platforms are excellent for developing technical knowledge, they do not cover all the competencies required in a genuine data project, where compromises, contextual factors, and assumptions must be critically evaluated.
Having reviewed numerous resumes, I can now easily spot when technical skills and projects lack the accompanying ability to apply them effectively in real-world scenarios. Consequently, I frequently advise aspiring data scientists to gain practical experience by engaging in projects with tangible applications—whether through volunteering, internships, or collaborations with small startups.
Chapter 2: Insights into Data Science Careers
In this video, "The Harsh Reality of Being a Data Scientist," we explore the challenges and misconceptions surrounding the field of data science.
"The Hard Parts of Data Science Made Easy" breaks down the complexities and provides strategies for overcoming them in your career.